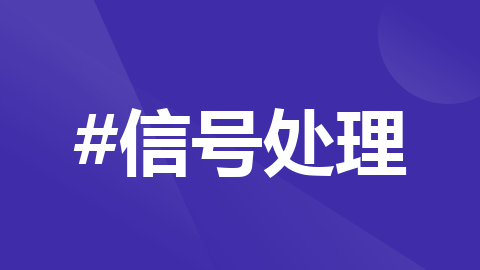
GPU实现雷达信号处理二维ca-cfar,cuda加速完整代码
【代码】GPU实现雷达信号处理二维ca-cfar,cuda加速完整代码。
·
二维ca-cfar cuda加速实现,完整代码如下,
导入输入数据即可,后期完善后补发更详细代码
// GPU cuda实现雷达信号处理cfar
#include<stdio.h>
#include <cuda.h>
#include"iostream"
#include"cuda_runtime_api.h"
#include"device_launch_parameters.h"
#include"cufft.h"
#include<math.h>
#include <string.h>
#include <cuda_runtime.h>
#include <windows.h>
__global__ void cfar_2d_kernel2(float* PC_data_ifft_CA_abs, int* location, float* threshold_F, int M_row, int N_point, int N_prot_F, int N_ref_F, float alpha) {
int i = blockIdx.x * blockDim.x + threadIdx.x;
int j = blockIdx.y * blockDim.y + threadIdx.y;
if (i < M_row && j < N_point) {
if (location[i * N_point + j] == 1) {
if (i <= (N_prot_F + N_ref_F)) { //观察点过于偏左,左侧点数不足
float sum_F = 0;
for (int k = i + N_prot_F + 1; k <= i + N_prot_F + N_ref_F; k++) {
sum_F += PC_data_ifft_CA_abs[k * N_point + j];
}
threshold_F[i * N_point + j] = sum_F / N_ref_F; //观察点右侧参考点相加求平均
}
else if (i >= (M_row - N_prot_F - N_ref_F )) { //观察点过于偏右,右侧点数不足
float sum_F = 0;
for (int k = i - N_prot_F - N_ref_F; k <= i - N_prot_F - 1; k++) {
sum_F += PC_data_ifft_CA_abs[k * N_point + j];
}
threshold_F[i * N_point + j] = sum_F / N_ref_F; //观察点左侧参考点求平均
}
else { //观察点居中,左右点数均足够
float sum_F_left = 0;
float sum_F_right = 0;
for (int k = i - N_prot_F - N_ref_F; k <= i - N_prot_F - 1; k++) {
sum_F_left += PC_data_ifft_CA_abs[k * N_point + j];
}
for (int k = i + N_prot_F + 1; k <= i + N_prot_F + N_ref_F; k++) {
sum_F_right += PC_data_ifft_CA_abs[k * N_point + j];
}
threshold_F[i * N_point + j] = fmax(sum_F_left, sum_F_right) / N_ref_F; //观察点两侧取大的一侧求均值
}
}
else {
threshold_F[i * N_point + j] = 0;
}
}
}
__global__ void cfar_2d_kernel(float* PC_data_ifft_CA_abs, int* location, float* threshold_R, int M_row, int N_point, int N_prot_R, int N_ref_R, float alpha) {
int i = blockIdx.x * blockDim.x + threadIdx.x;
int j = blockIdx.y * blockDim.y + threadIdx.y;
if (i < M_row && j < N_point) {
if (location[i * N_point + j] == 1) {
if (j <= (N_prot_R + N_ref_R)) {
float sum_R = 0;
for (int k = j + N_prot_R + 1; k <= j + N_prot_R + N_ref_R; k++) {
sum_R += PC_data_ifft_CA_abs[i * N_point + k];
}
threshold_R[i * N_point + j] = sum_R / N_ref_R * alpha;
}
else if (j >= (N_point - N_prot_R - N_ref_R)) {
float sum_R = 0;
for (int k = j - N_prot_R - N_ref_R; k <= j - N_prot_R - 1; k++) {
sum_R += PC_data_ifft_CA_abs[i * N_point + k];
}
threshold_R[i * N_point + j] = (sum_R / N_ref_R) * alpha;
}
else {
float sum_R_left = 0;
float sum_R_right = 0;
for (int k = j - N_prot_R - N_ref_R; k <= j - N_prot_R - 1; k++) {
sum_R_left += PC_data_ifft_CA_abs[i * N_point + k];
}
for (int k = j + N_prot_R + 1; k <= j + N_prot_R + N_ref_R; k++) {
sum_R_right += PC_data_ifft_CA_abs[i * N_point + k];
}
threshold_R[i * N_point + j] = (sum_R_left + sum_R_right) / (N_ref_R * 2) * alpha;
}
}
else {
threshold_R[i * N_point + j] = 0;
}
}
}
//void cfar_2d(float* PC_data_ifft_CA_abs, int* location, float* threshold_R, int M_row, int N_point, int N_prot_R, int N_ref_R, float alpha) {
// int block_size = 16;
// dim3 threadsPerBlock(block_size, block_size);
// dim3 numBlocks((M_row + threadsPerBlock.x - 1) / threadsPerBlock.x, (N_point + threadsPerBlock.y - 1) / threadsPerBlock.y);
// cfar_2d_kernel << <numBlocks, threadsPerBlock >> > (PC_data_ifft_CA_abs, location, threshold_R, M_row, N_point, N_prot_R, N_ref_R, alpha);
//}
__global__ void calc_sum_ref_2D(float* d_PC_data_ifft_CA_abs, float* d_sum_ref_2D, const int N_point, const int N_ref_2D, const int M) {
int idx = blockIdx.x * blockDim.x + threadIdx.x; //获取当前线程的全局唯一ID
if (idx == 0) {
d_sum_ref_2D[0] = 0;
d_sum_ref_2D[1] = 0;
d_sum_ref_2D[2] = 0;
d_sum_ref_2D[3] = 0;
}
//__syncthreads(); //保证所有线程都执行完上一步操作
//计算区域1的和
if (idx < N_ref_2D * N_ref_2D) {
int i = idx / N_ref_2D;
int j = idx % N_ref_2D;
float sum1 = d_PC_data_ifft_CA_abs[i * N_point + j];
atomicAdd(&d_sum_ref_2D[0], sum1);
}
//计算区域2的和
if (idx < N_ref_2D * N_ref_2D) {
int i = idx / N_ref_2D;
int j = idx % N_ref_2D;
float sum2 = d_PC_data_ifft_CA_abs[i * N_point + N_point - N_ref_2D + j];
atomicAdd(&d_sum_ref_2D[1], sum2);
}
//计算区域3的和
if (idx < N_ref_2D * N_ref_2D) {
int i = M / 2 - N_ref_2D + idx / N_ref_2D;
int j = idx % N_ref_2D;
float sum3 = d_PC_data_ifft_CA_abs[i * N_point + j];
atomicAdd(&d_sum_ref_2D[2], sum3);
}
//计算区域4的和
if (idx < N_ref_2D * N_ref_2D) {
int i = M / 2 - N_ref_2D + idx / N_ref_2D;
int j = idx % N_ref_2D;
float sum4 = d_PC_data_ifft_CA_abs[i * N_point + N_point - N_ref_2D + j];
atomicAdd(&d_sum_ref_2D[3], sum4);
}
}
int main() {
LARGE_INTEGER frequency1, frequency2, frequency3, start_time1, end_time1, start_time2, end_time2, start_time3, end_time3, start_time4, end_time4, start_time5, end_time5;
double elapsed_time1, elapsed_time2, elapsed_time3, elapsed_time4, elapsed_time5, elapsed_time;
int const M = 64, N = 128; //M/2=8为行数 , N_ref_2D=4为参考窗长度,应该为N_point的一半, N_point=8为列数
int const N_ref_2D = 8;
int const N_point = 1080; //二维数组只认常数const
//*************动态存储**********************
float* PC_data_ifft_CA_abs = NULL; // 定义指向float类型的指针
cudaMallocHost((void**)&PC_data_ifft_CA_abs,32 * 1080 * sizeof(float));
int size = 0; // 定义数组长度变量
int i = 0; // 计数器
FILE* fp = fopen("F:/A-雷达信号处理/MATLAB的雷达数字信号处理/基于MATLAB的雷达数字信号处理/b.txt", "r"); // 打开文件
if (fp == NULL) { // 判断文件是否打开成功
printf("文件打开失败!\n");
return 1; // 返回错误码1表示程序异常结束
}
while (fscanf(fp, "%*f") != EOF) { // 统计文件中的数据数量
size++; // 数组长度加1
}
PC_data_ifft_CA_abs = (float*)malloc(size * sizeof(float)); // 动态分配数组内存空间
if (PC_data_ifft_CA_abs == NULL) { // 判断内存是否分配成功
printf("内存分配失败!\n");
return 1; // 返回错误码1表示程序异常结束
}
rewind(fp); // 将文件指针重新指向文件开头
while (fscanf(fp, "%f", &PC_data_ifft_CA_abs[i]) != EOF && i < size) { // 读取文件中的数据到数组中
i++; // 计数器加1
}
fclose(fp); // 关闭文件
/*
for (int j = 0; j < i; j++) { // 遍历数组输出内容
printf("%f\t", PC_data_ifft_CA_abs[j]);
}*/
// *************************************************** 2d筛选 ********************************************
//在主函数中调用
float sum_ref_2D[1][4] = { 0 }; //定义二维数组,存放参考区域的和
float *d_PC_data_ifft_CA_abs, *d_sum_ref_2D;
cudaMalloc((void**)&d_PC_data_ifft_CA_abs, 32 * N_point * sizeof(float));
cudaMemcpy(d_PC_data_ifft_CA_abs, PC_data_ifft_CA_abs, 32 * N_point * sizeof(float), cudaMemcpyHostToDevice);
cudaMalloc((void**)&d_sum_ref_2D, 4 * sizeof(float));
calc_sum_ref_2D << <1, N_ref_2D* N_ref_2D >> > (d_PC_data_ifft_CA_abs, d_sum_ref_2D, N_point, N_ref_2D, M);
cudaMemcpy(sum_ref_2D, d_sum_ref_2D, 4 * sizeof(float), cudaMemcpyDeviceToHost);
//cudaFree(d_PC_data_ifft_CA_abs); //释放显存
cudaFree(d_sum_ref_2D); //释放显存
printf("\n%f + %f + %f + %f\n", sum_ref_2D[0][0], sum_ref_2D[0][1], sum_ref_2D[0][2], sum_ref_2D[0][3]);
float SNR_Threshold = 15;
//产生门限Threshold
float Threshold = 0;
float sum = 0;
for (int i = 0; i < 4; i++) {
sum += sum_ref_2D[0][i];
}
printf("sum = %f\n", sum);
printf("min = %f\n", fminf(fminf(sum_ref_2D[0][0], sum_ref_2D[0][1]), fminf(sum_ref_2D[0][2], sum_ref_2D[0][3])));
printf("min1 = %f\n", ((sum - fminf(fminf(sum_ref_2D[0][0], sum_ref_2D[0][1]), fminf(sum_ref_2D[0][2], sum_ref_2D[0][3]))) / (N_ref_2D * N_ref_2D * 3)));
printf("min2= %f\n", powf(10, SNR_Threshold / 10));
if (SNR_Threshold >= 15) {
Threshold = ((sum - fminf(fminf(sum_ref_2D[0][0], sum_ref_2D[0][1]), fminf(sum_ref_2D[0][2], sum_ref_2D[0][3]))) / (N_ref_2D * N_ref_2D * 3)) * powf(10, SNR_Threshold / 10);
}
else if (SNR_Threshold <= 3) {
Threshold = ((sum - fmaxf(fmaxf(sum_ref_2D[0][0], sum_ref_2D[0][1]), fmaxf(sum_ref_2D[0][2], sum_ref_2D[0][3]))) / (N_ref_2D * N_ref_2D * 3)) * powf(10, SNR_Threshold / 10);
}
else {
float max_sum = fmaxf(fmaxf(sum_ref_2D[0][0], sum_ref_2D[0][1]), fmaxf(sum_ref_2D[0][2], sum_ref_2D[0][3]));
float min_sum = fminf(fminf(sum_ref_2D[0][0], sum_ref_2D[0][1]), fminf(sum_ref_2D[0][2], sum_ref_2D[0][3]));
Threshold = ((sum - max_sum - min_sum) / (N_ref_2D * N_ref_2D * 2)) * powf(10, SNR_Threshold / 10);
}
printf("Threshold = %f\n", Threshold);
//寻找PC_data_ifft_CA中大于门限的点并记录其位置
int* location = NULL; // 定义指向float类型的指针
cudaMallocHost((void**)&location, 32 * 1080 * sizeof(float));
int const M_row = M / 2;
// int location[M_row][N_point];
// 将location数组中的所有元素设置为0
/* for (int i = 0; i < M_row; i++) {
for (int j = 0; j < N_point; j++) {
location[i][j] = 0;
}
}*/
for (int i = 0; i < M_row; i++) {
for (int j = 0; j < N_point; j++) {
if (PC_data_ifft_CA_abs[i * N_point + j] >= (1 * Threshold)) {
location[i * N_point + j] = 1;
printf("i=%d\t j=%d\n", i, j);
printf("%f\n", PC_data_ifft_CA_abs[i * N_point + j]);
}
else {
location[i * N_point + j] = 0;
}
}
}
//***********输出********************************
/* for (int i = 0; i < M_row; i++) {
for (int j = 0; j < N_point; j++) {
printf("%d\t",location[i][j]);
}
printf("\n");
}*/
//一维距离筛选 cuda语言 *************************************************** 1.1 *********************************************
int N_prot_R = 30; //保护单元覆盖第二旁瓣,次大点与次次大点
int N_ref_R = 135; //参考单元
float alpha = 3; // ------门限因子
float* threshold_R = NULL;
cudaMallocHost((void**)&threshold_R, 32 * 1080 * sizeof(float));
float* dev_threshold_R;
int* dev_location;
cudaMalloc((void**)&dev_location, M_row * N_point * sizeof(int));
cudaMalloc((void**)&dev_threshold_R, M_row * N_point * sizeof(float));
cudaMemcpy(dev_location, location, M_row * N_point * sizeof(int), cudaMemcpyHostToDevice);
int block_size = 16;
dim3 threadsPerBlock(block_size, block_size); //block_size * block_size个线程
dim3 numBlocks((M_row + threadsPerBlock.x - 1) / threadsPerBlock.x, (N_point + threadsPerBlock.y - 1) / threadsPerBlock.y); //正好包含所有数据
//-------------------------------------------------------time 1--------------------------------------------
QueryPerformanceFrequency(&frequency1);
QueryPerformanceCounter(&start_time1);
cfar_2d_kernel << <numBlocks, threadsPerBlock >> > (d_PC_data_ifft_CA_abs, dev_location, dev_threshold_R, M_row, N_point, N_prot_R, N_ref_R, alpha);
QueryPerformanceCounter(&end_time1);
elapsed_time1 = (double)(end_time1.QuadPart - start_time1.QuadPart) / frequency1.QuadPart;
//-----------------------------------------------------------------------------------------------------
cudaMemcpy(threshold_R, dev_threshold_R, M_row* N_point * sizeof(float), cudaMemcpyDeviceToHost);
//*********输出 threshold_R *****
printf("\nthreshold_R[i][j]: \n");
for (int i = 0; i < M_row; i++) {
for (int j = 0; j < N_point; j++) {
if (location[i * N_point + j] == 1) {
printf("%f\t", threshold_R[i * N_point + j]);
printf("%d+%d\n", i, j);
}
}
}
//利用距离维门限进行一维筛选*************************************************** 1.2 *********************************************
int* location_R = NULL;
cudaMallocHost((void**)&location_R, 32 * 1080 * sizeof(float));
// int location_R[M_row * N_point] = { 0 }; //利用距离维门限进行一维筛选
for (int i = 0; i < M_row; i++) {
for (int j = 0; j < N_point; j++) {
if (location[i * N_point + j] == 1) { //只筛选经过二维筛选之后的点
if (PC_data_ifft_CA_abs[j + i * N_point] >= (1 * threshold_R[i * N_point + j])) {
location_R[i * N_point + j] = 1;
}
else {
location_R[i * N_point + j] = 0;
}
}
else {
location_R[i * N_point + j] = 0;
}
}
}
/*
printf("\nlocation_R[i][j]: \n");
for (int i = 0; i < M_row; i++) {
for (int j = 0; j < N_point; j++) {
printf("%d\t", location_R[i][j]);
}
printf("\n");
}*/
2 频率维筛选 cuda语言*************************************************** 2.1 *********************************************
int N_prot_F = 5;
int N_ref_F = 10;
// float threshold_F[M_row][N_point] = { 0 }; //记录距离维每个观察点的门限值
float* threshold_F = NULL; // 定义指向float类型的指针
cudaMallocHost((void**)&threshold_F, 32 * 1080 * sizeof(float));
int* dev_location_R;
float* dev_threshold_F;
cudaMalloc((void**)&dev_location_R, M_row * N_point * sizeof(int));
cudaMalloc((void**)&dev_threshold_F, M_row * N_point * sizeof(float));
cudaMemcpy(dev_location_R, location_R, M_row * N_point * sizeof(int), cudaMemcpyHostToDevice);
int block_size2 = 16;
dim3 threadsPerBlock2(block_size2, block_size2);
dim3 numBlocks2((M_row + threadsPerBlock.x - 1) / threadsPerBlock.x, (N_point + threadsPerBlock.y - 1) / threadsPerBlock.y);
//-------------------------------------------------------time 2--------------------------------------------
QueryPerformanceFrequency(&frequency2);
QueryPerformanceCounter(&start_time2);
cfar_2d_kernel2 << <numBlocks2, threadsPerBlock2 >> > (d_PC_data_ifft_CA_abs, dev_location_R, dev_threshold_F, M_row, N_point, N_prot_F, N_ref_F, alpha);
QueryPerformanceCounter(&end_time2);
elapsed_time2 = (double)(end_time2.QuadPart - start_time2.QuadPart) / frequency2.QuadPart;
cudaMemcpy(threshold_F, dev_threshold_F, M_row* N_point * sizeof(float), cudaMemcpyDeviceToHost);
cudaFree(d_PC_data_ifft_CA_abs); //释放显存
elapsed_time = elapsed_time1 + elapsed_time2 ;
printf("elapsed_time1: %f ms elapsed_time2: %f ms \n",(elapsed_time1 * 1000), (elapsed_time2 * 1000));
printf("GPU运行时间: %f ms\n", (elapsed_time * 1000));
/*printf("\nthreshold_F[i][j]: \n");
for (int i = 0; i < M_row; i++) {
for (int j = 0; j < N_point; j++) {
printf("%f\t", threshold_F[i][j]);
}
printf("\n");
}*/
//%利用频率维门限进行一维筛选 cuda语言 *************************************************** 2.2 *********************************************
int location_R_F[M_row][N_point] = { 0 }; //利用频率维门限进行一维筛选
for (int i = 0; i < M_row; i++) {
for (int j = 0; j < N_point; j++) {
if (location_R[i * N_point + j] == 1) { //只筛选经过二维筛选和距离维筛选之后的点
if (PC_data_ifft_CA_abs[j + i * N_point] >= (1 * threshold_F[i * N_point + j])) {
location_R_F[i][j] = 1;
printf("i=%d\t j=%d\n", i, j);
printf("%f\n", PC_data_ifft_CA_abs[i * N_point + j]);
}
else {
location_R_F[i][j] = 0;
}
}
else {
location_R_F[i][j] = 0;
}
}
}
/*printf("\nlocation_R_F[i][j]: \n");
for (int i = 0; i < M_row; i++) {
for (int j = 0; j < N_point; j++) {
printf("%d\t", location_R_F[i][j]);
}
printf("\n");
}*/
free(PC_data_ifft_CA_abs); // 释放数组内存空间
return 0;
}
更多推荐
所有评论(0)